A Novel Detection Strategy for Kidnapped Robot Problem in Monte Carlo Localization Using Corridor-Type Map
Abstract
Full Text:
PDFReferences
S. Thrun, W. Burgard and D. Fox, Probabilistic Robotics (MIT Press, Cambridge, MA, 2005).
L. Zhang, R. Zapata and P. Lépinay, “Self-adaptive Monte Carlo localization for mobile robots using range finders,” Robotica 30, pp.229–244 (2012).
Yi, C. and B.-U. Choi, Detection and Recovery for Kidnapped-Robot Problem Using Measurement Entropy, in Grid and Distributed Computing, T.-h. Kim, et al., Editors. 2011, Springer Berlin Heidelberg. p. 293-299.
Majdik, Andras, et al. "New approach in solving the kidnapped robot problem."Robotics (ISR), 2010 41st International Symposium on and 2010 6th German Conference on Robotics (ROBOTIK). VDE, 2010.
Andreasson, Henrik, André Treptow, and Tom Duckett. "Localization for mobile robots using panoramic vision, local features and particle filter." Robotics and Automation, 2005. ICRA 2005. Proceedings of the 2005 IEEE International Conference on. IEEE, 2005.
Duan, Z.; Cai, Z.; Min, H. Robust Dead Reckoning System for Mobile Robots Based on Particle Filter and Raw Range Scan. Sensors 2014, 14, 16532–16562.
T. Hester and P. Stone, “Negative Information and Line Observations for Monte-Carlo Localization,” Proceedings of IEEE International Conference on Robotics and Automation ICRA 2008, Pasadena, CA (2008) pp. 2764-2769.
S. Thrun, D. Fox, W. Burgard and F. Dellaert, “Robust Monte Carlo Localization for Mobile Robots,” Artif. Intell. 128(1-2), 99-141(2001).
H. Andreasson, A. Treptow and T. Duckett, “Localization for Mobile Robots Using Panoramic Vision, Local Features and Particle Filter,” Proceeding of IEEE International Conference on Robotics and Automation (ICRA 05), Barcelona, Spain (2005) pp. 3348-3353.
A. R. Vahdat, N. NourAshrafoddin and S. S. Ghidary, “Mobile Robot Global Localization Using Differential Evolution and Particle Swarm Optimization,” IEEE Congress on Evolutionary Computation (CEC 07), Singapore (2007) pp. 1527-1534.
S. I. Roumeliotis and G. A. Bekey, “Bayesian Estimation and Kalman Filtering: A Unified Framework for Mobile Robot Localization,” Proceedings of IEEE International Conference on Robotics and Automation (ICRA ’00), San Francisco, CA (2000) vol.3, pp. 2985-2992.
S. Thrun, M. Beetz, M. Bennewitz, W. Buggard, A. Cremers, F. Dellaert, D. Fox, D. Hähnel, C. Rosenberg, N. Roy, J. Schulte, and D. Schulz, “Probabilistic Algorithms and The Interactive Museum Tour-Guide Robot Minerva,” Int. J. Robot. Res. 19, 972-999(2000).
L. Marchetti, G. Grisetti, and L. Iocchi, “A Comparative Analysis of Particle Filter Based Localization Methods,” RoboCup, Bremen, Germany (2006), pp. 442-449.
D. Fox, W. Burgard, H. Kruppa, and S. Thrun, “Efficient Multi-Robot Localization Based on Monte Carlo Approximation,” In Robotic Research: The Ninth International Symposium (J. Hollerbach and D. Koditschek, Eds.) (Springer-Verlag, London, 2000).
C. Kwok, D. Fox and M. Meila, “Real-Time Particle Filters,” Proceedings of the IEEE, vol. 92, no. 3, pp. 469-484, 2004.
P. Pfaff, W. Burgard and D. Fox, “Robust Monte-Carlo Localization Using Adaptive Likelihood Models,” European Robotics Symposium, Palermo, Italy (SpringerVerlag, 2006) pp. 181-194.
C. Siagian and L. Itti, “Biologically-Inspired Robotics Vision Monte-Carlo Localization in the Outdoor Environment,” Proceedings of IEEE/RSJ International Conference on Intelligent Robots and Systems IROS 2007, San Diego, CA, (2007) pp. 1723-1730.
S. Thrun, D. Fox, and W. Burgard, “Monte Carlo localization with mixture proposal distribution,” in Proceedings of the AAAI National Conference on Artificial Intelligence, 2000, pp. 859–865.
I. Bukhori, Z.H. Ismail, and T. Namerikawa, “Detection Strategy for Kidnapped Robot Problem in Landmark-Based Map Monte Carlo Localization,” in IEEE International Symposium on Robotics and Intelligent Sensors 2015.
DOI: http://dx.doi.org/10.33021/jeee.v2i1.706
Refbacks
- There are currently no refbacks.
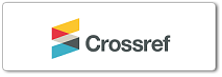
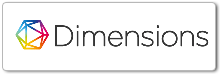
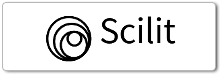
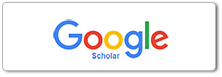